Energy efficiency maximization for full duplex MIMO cloud radio access networks
This paper studies the energy efficiency (EE) in full-duplex (FD) multiple-input multiple-output
(MIMO) cloud radio access networks (CRANs). A cloud control unit (CU) transfers information signals
with multiple downlink (DL) and uplink (UL) users through FD radio units (RUs) by limited capacity
FH links. In DL transmission, the signals intended to the DL users are centrally processed at the
CU and, then, compressed to transfer to the RUs before they are forwarded to the DL users. On
the other hand, the signals from the UL users to the RUs are compressed and forwarded to the
CU for signal detection processing. Thus, the precoding designs and compression strategies for
the UL and DL transmission are critical for the system performance. The conventional methods
commonly focus on maximizing the spectral efficiency (SE) in the networks. Although the SE maximization based methods can offer the superior SE performance, they can result in the inefficient
usage of the energy in the networks. Thus, this paper focuses on the joint design of the precoders
and quantization covariance matrices to maximize the EE. The EE maximization problem is formulated as an optimization problem of the precoders and quantization covariance matrices subject to
the transmit power constraints at each RU, each user and the limited capacity of FH links. To deal
with the non-convexity of the formulated design problem, we exploit a successive convex approximation (SCA) method to derive the concave lower bound function of the sum rate and a convex
upper bound of capacity functions of FH links. Then, the Dinkelbach approach is exploited to derive an efficient iterative algorithm (IA) guaranteeing convergence. Numerical results are given to
demonstrate the EE performance of the proposed algorithm.
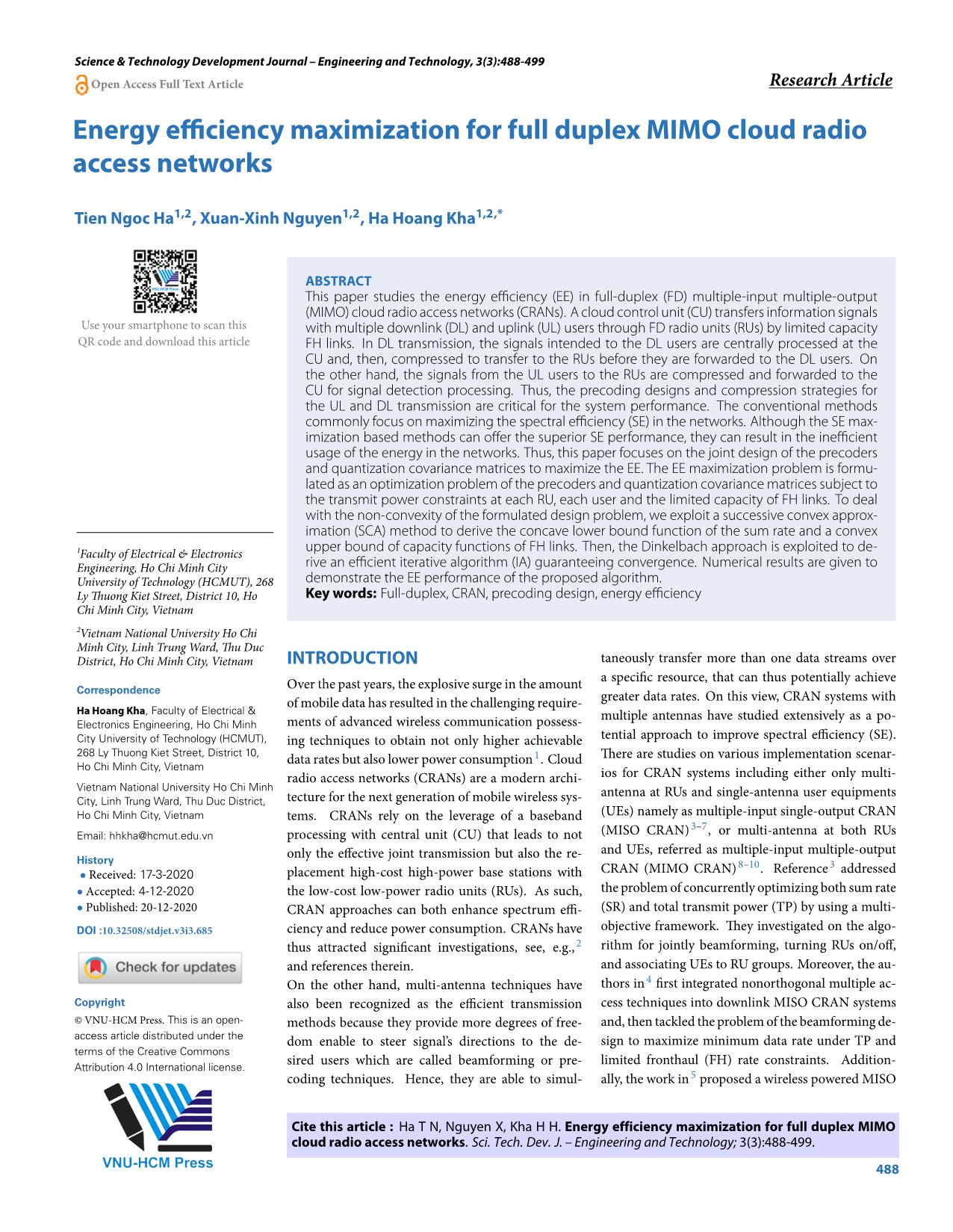
Trang 1
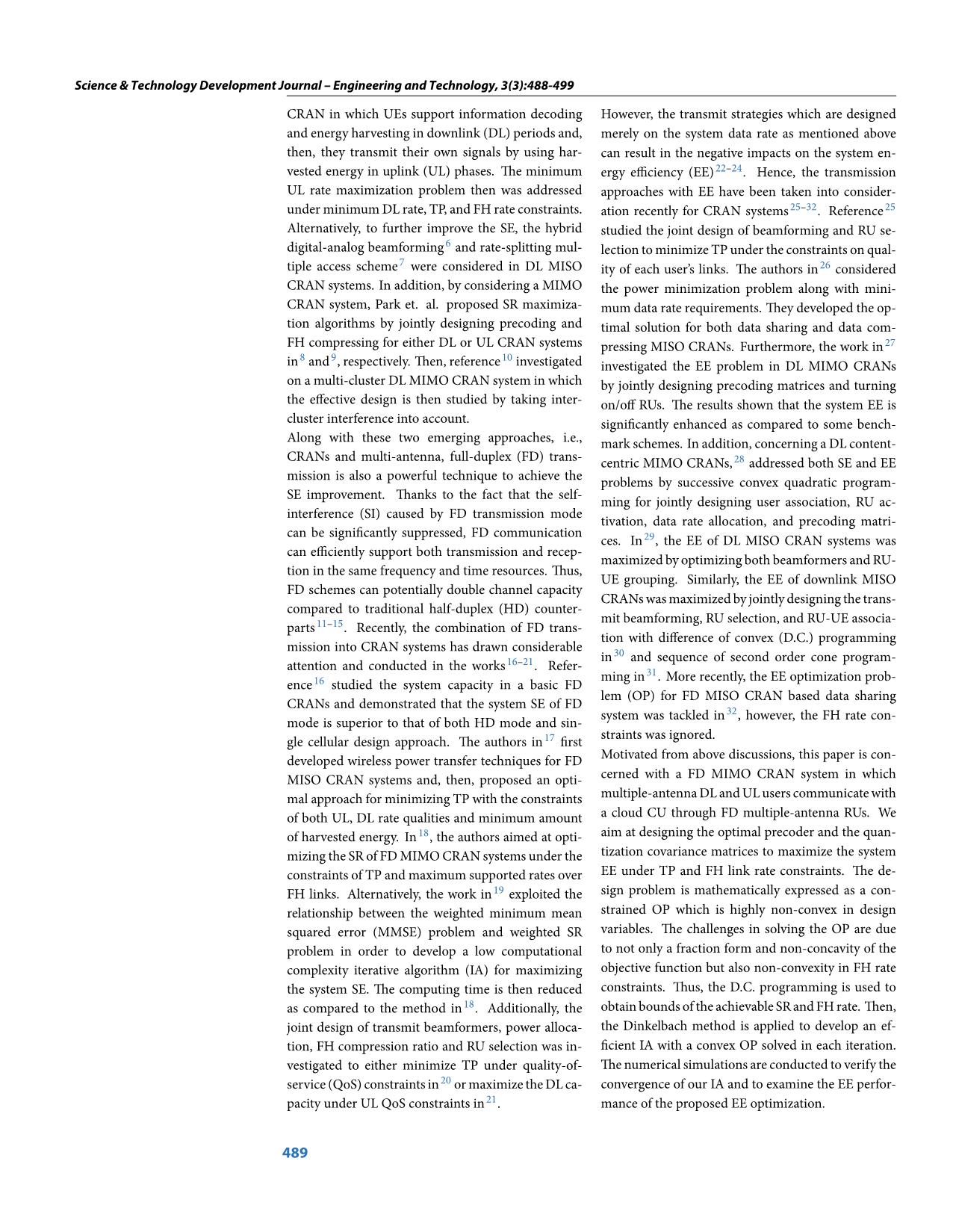
Trang 2
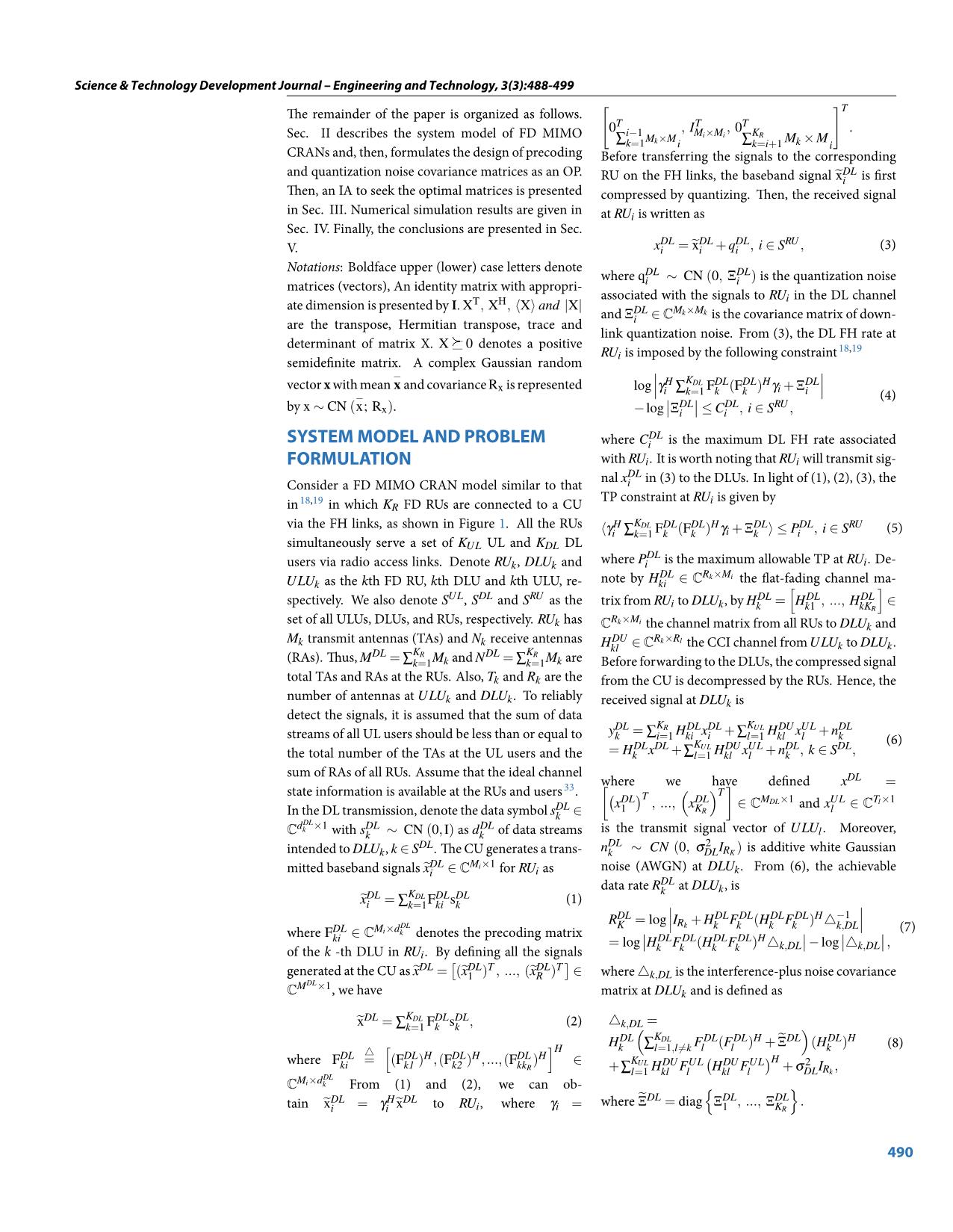
Trang 3
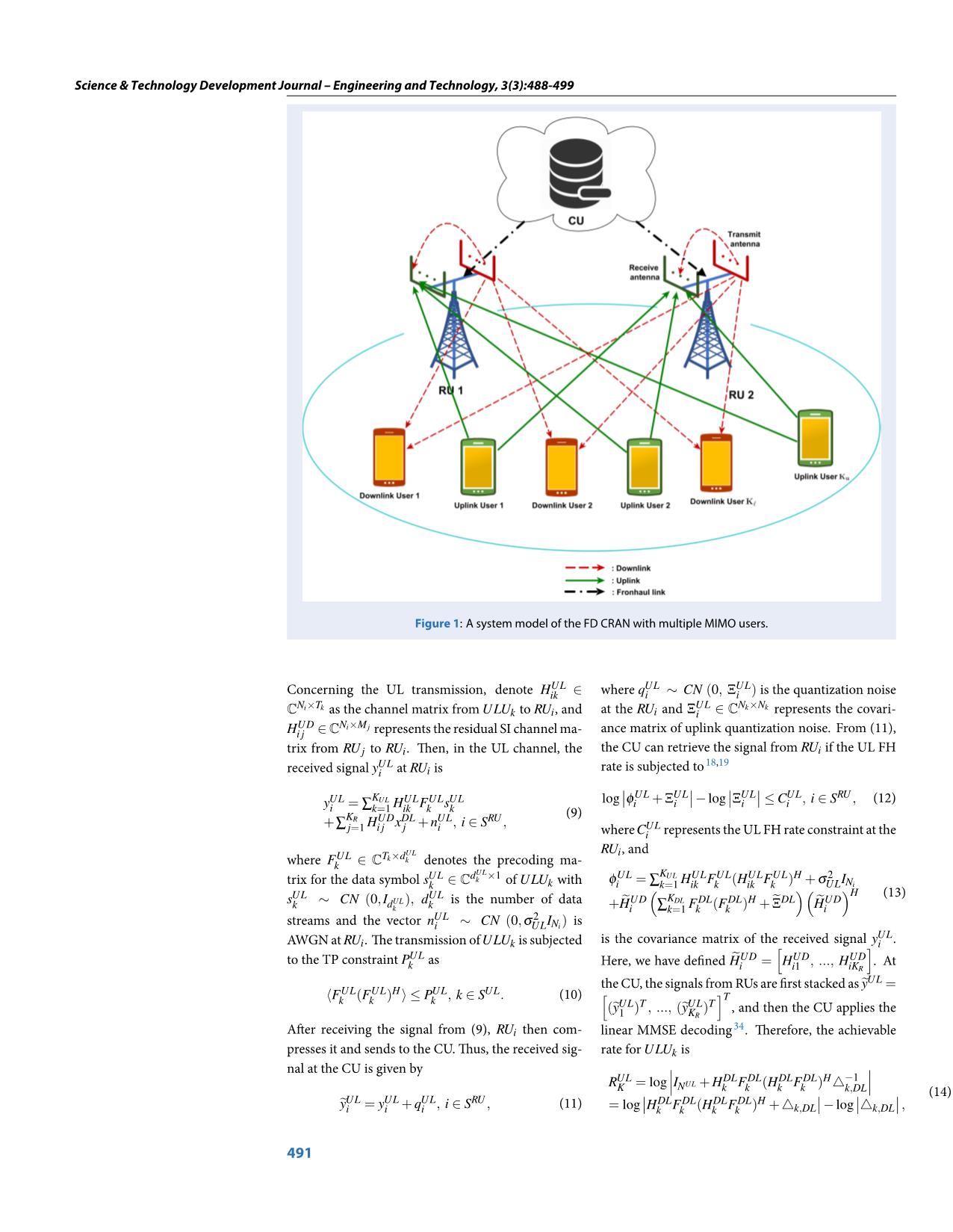
Trang 4
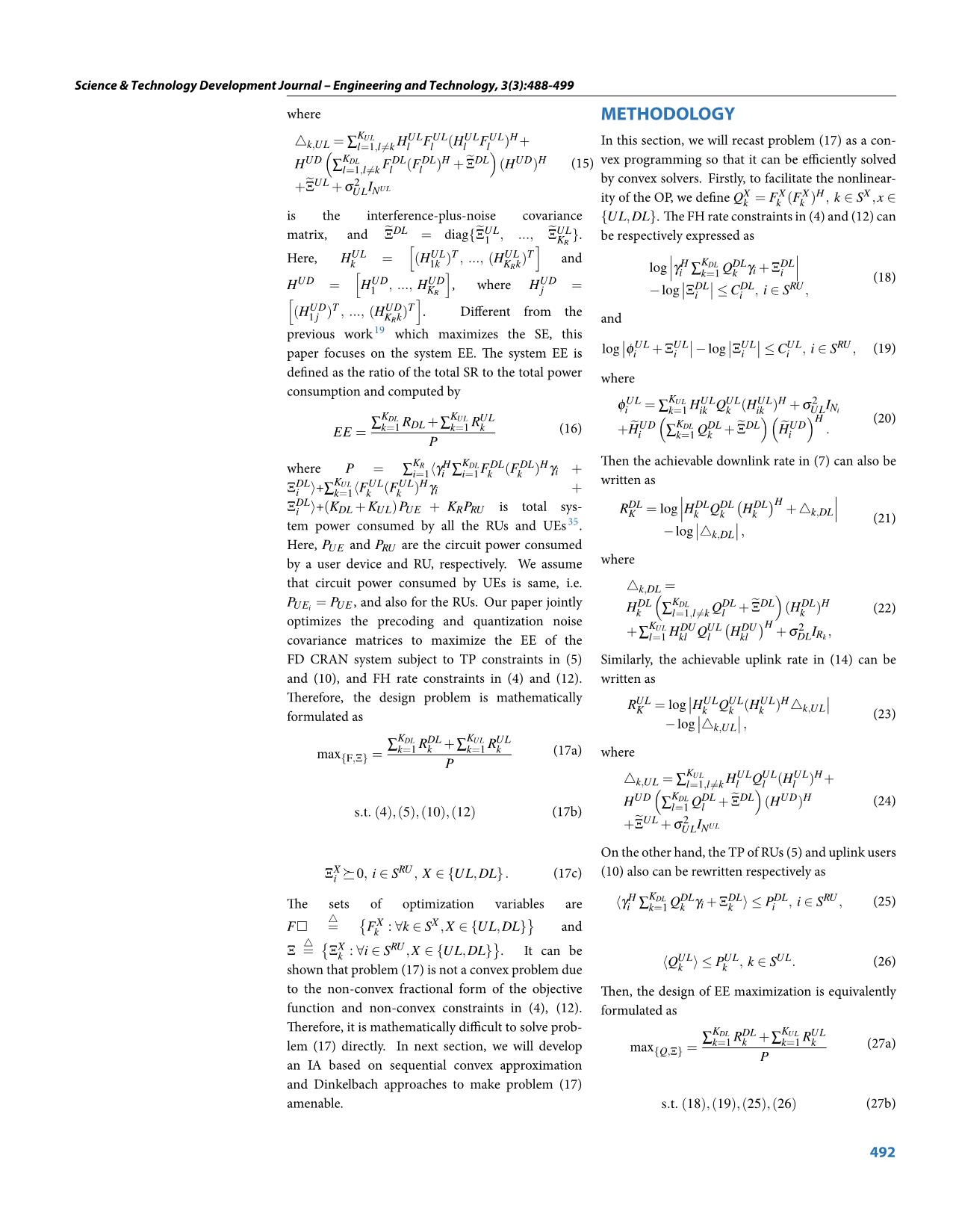
Trang 5
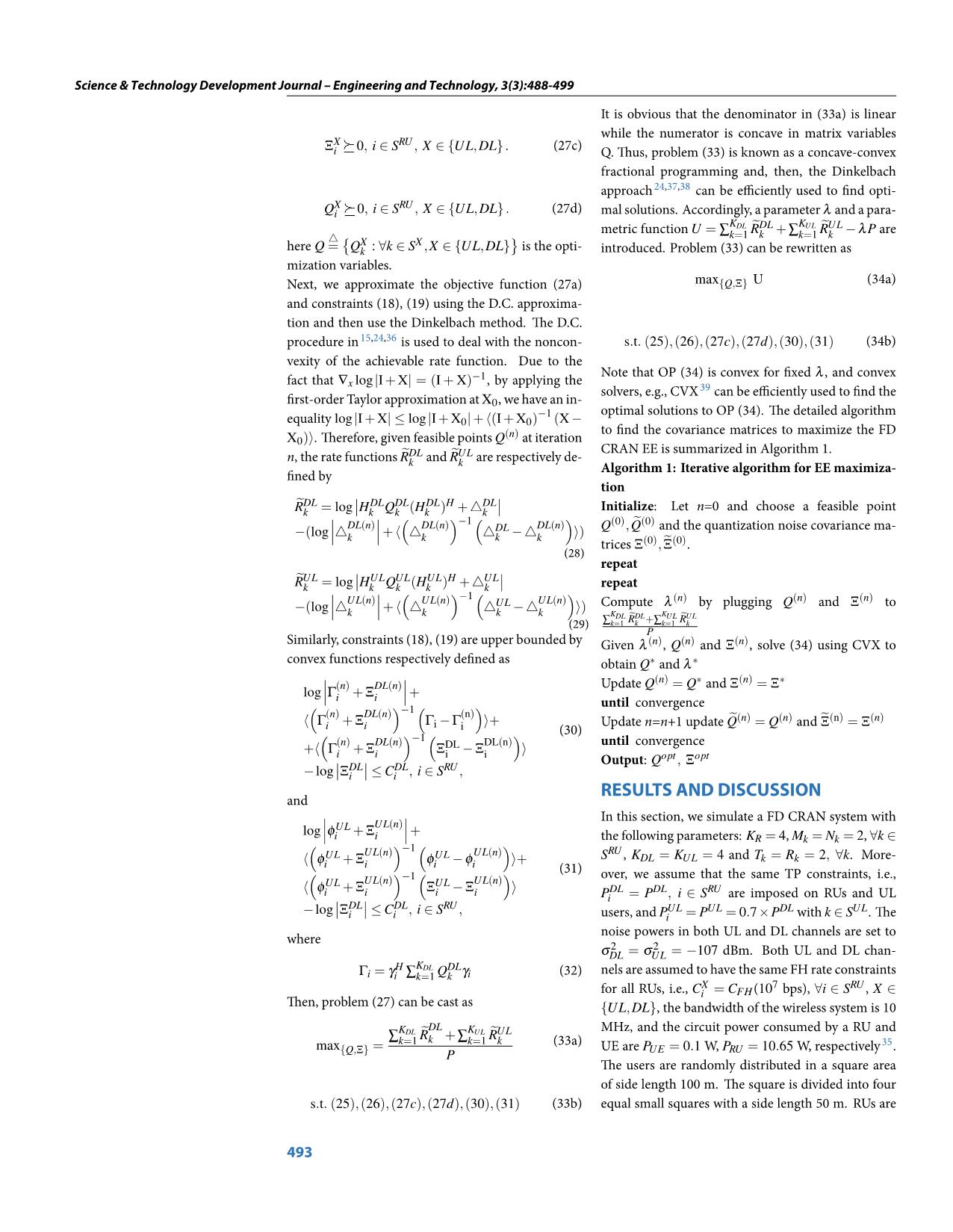
Trang 6
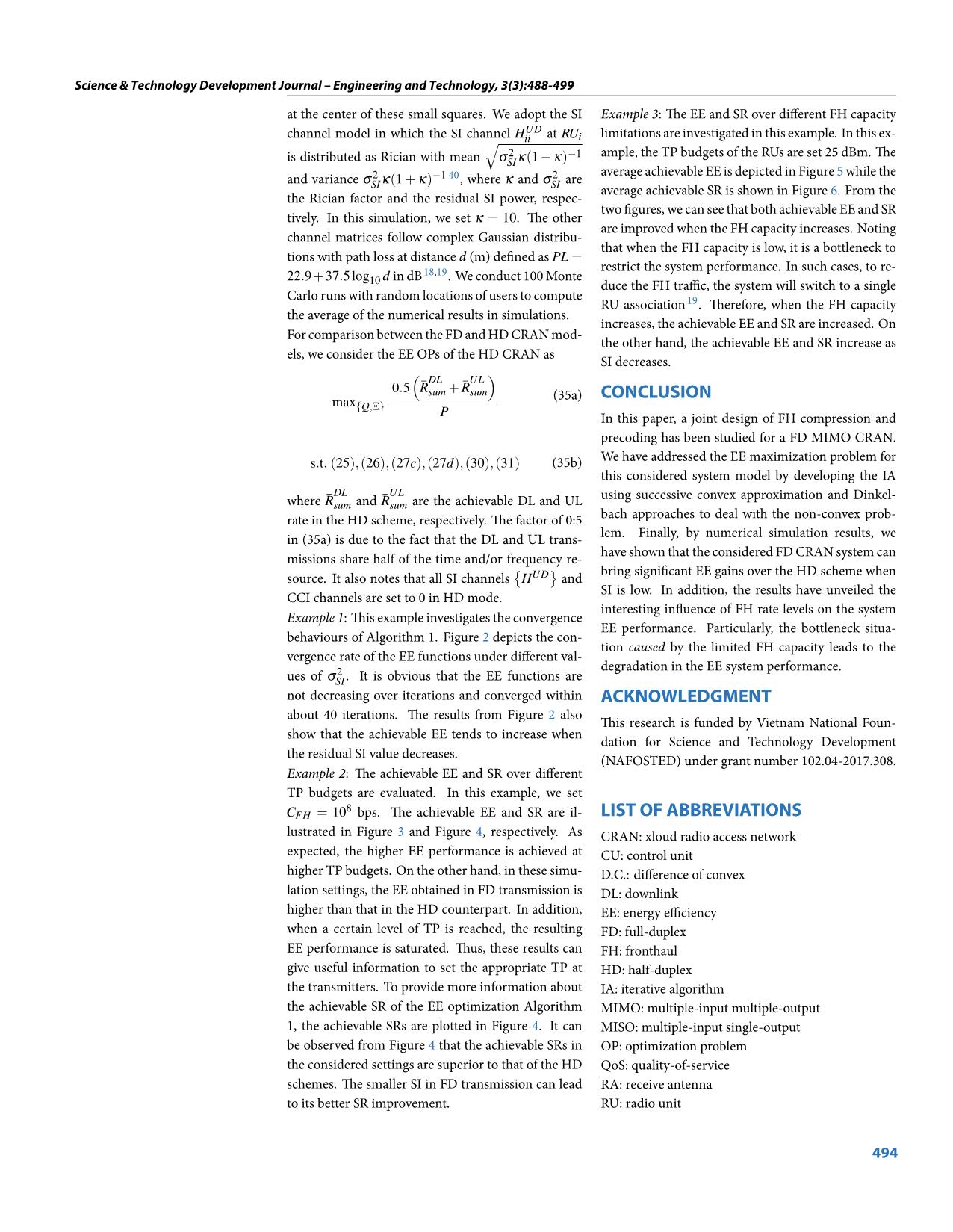
Trang 7
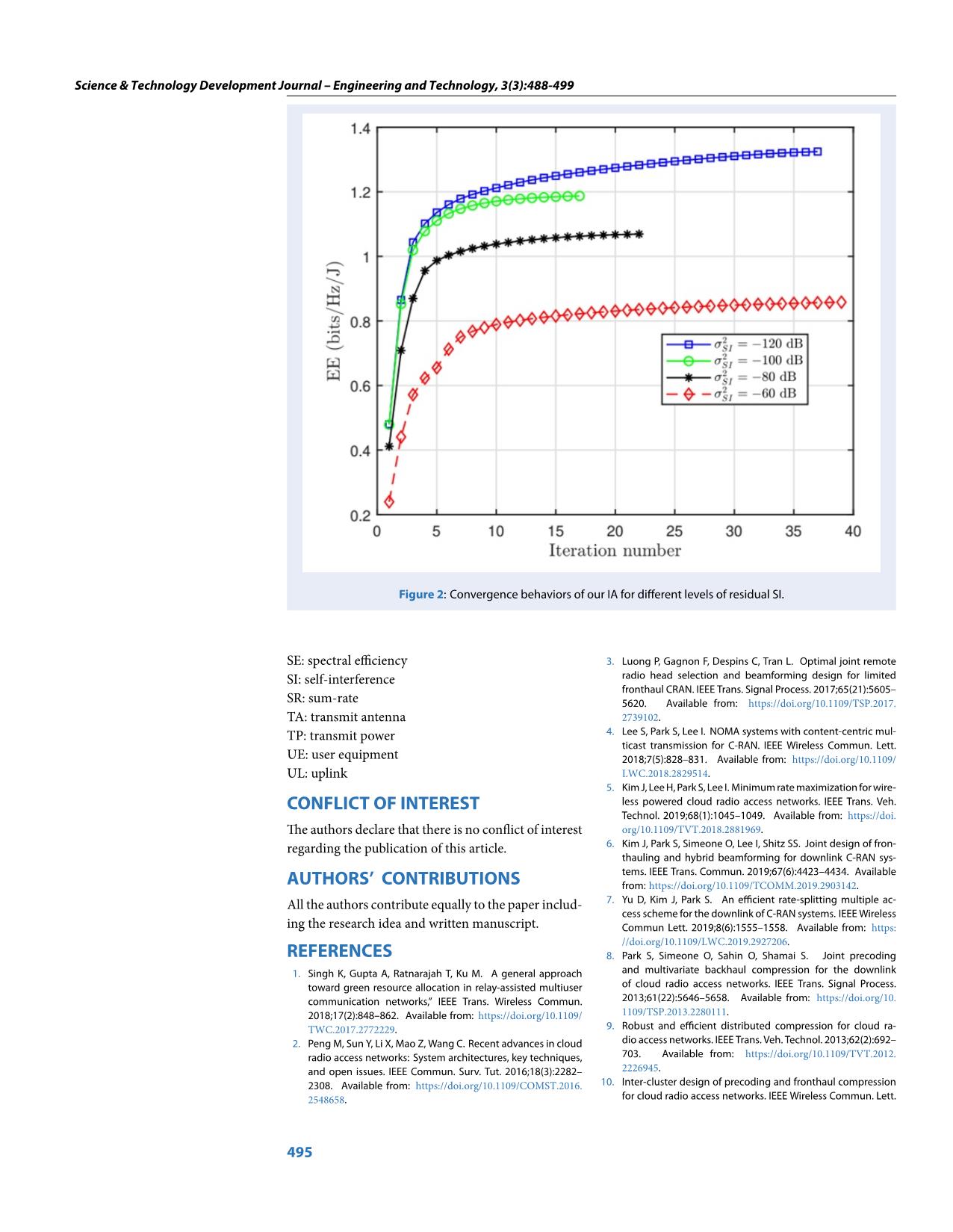
Trang 8
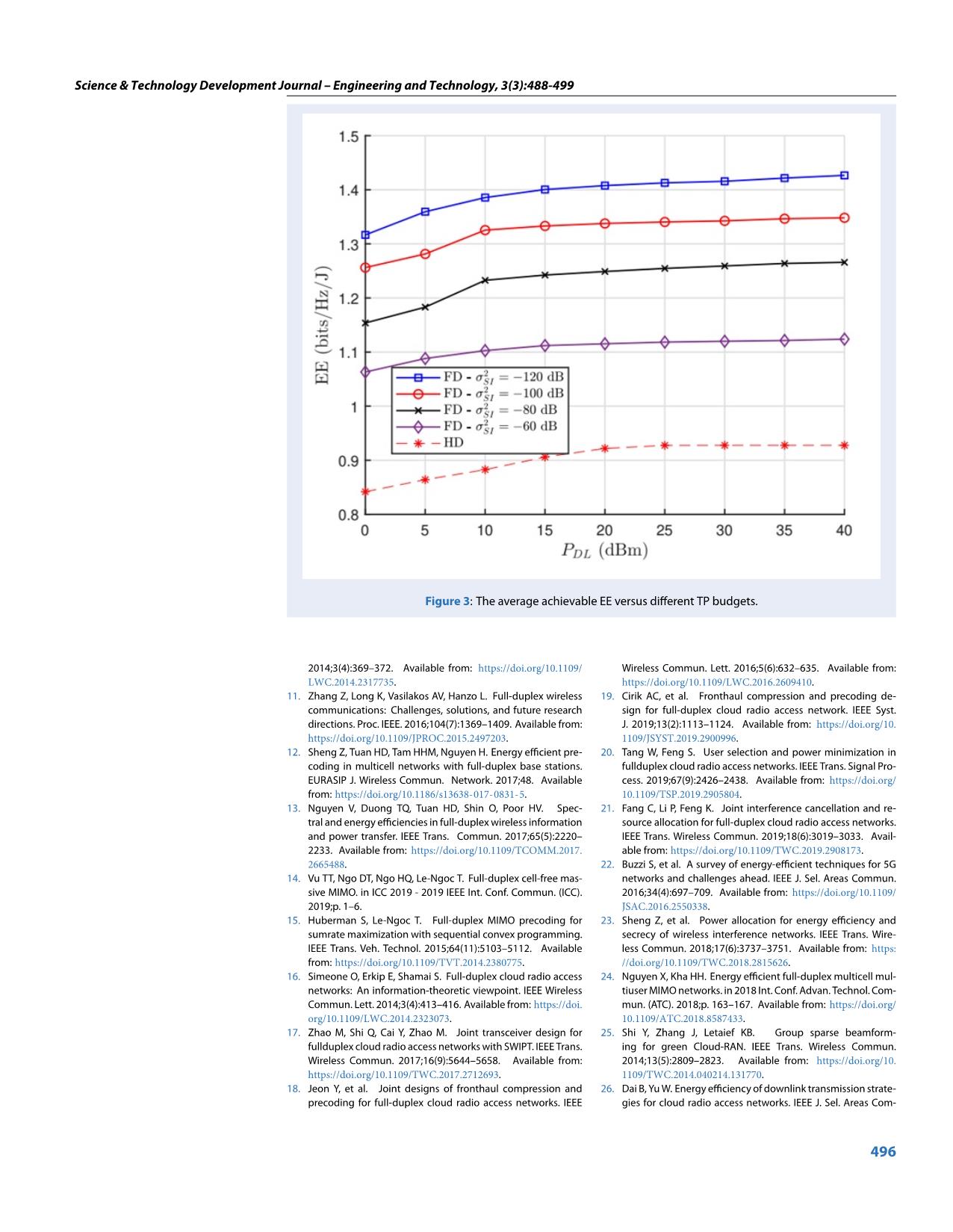
Trang 9
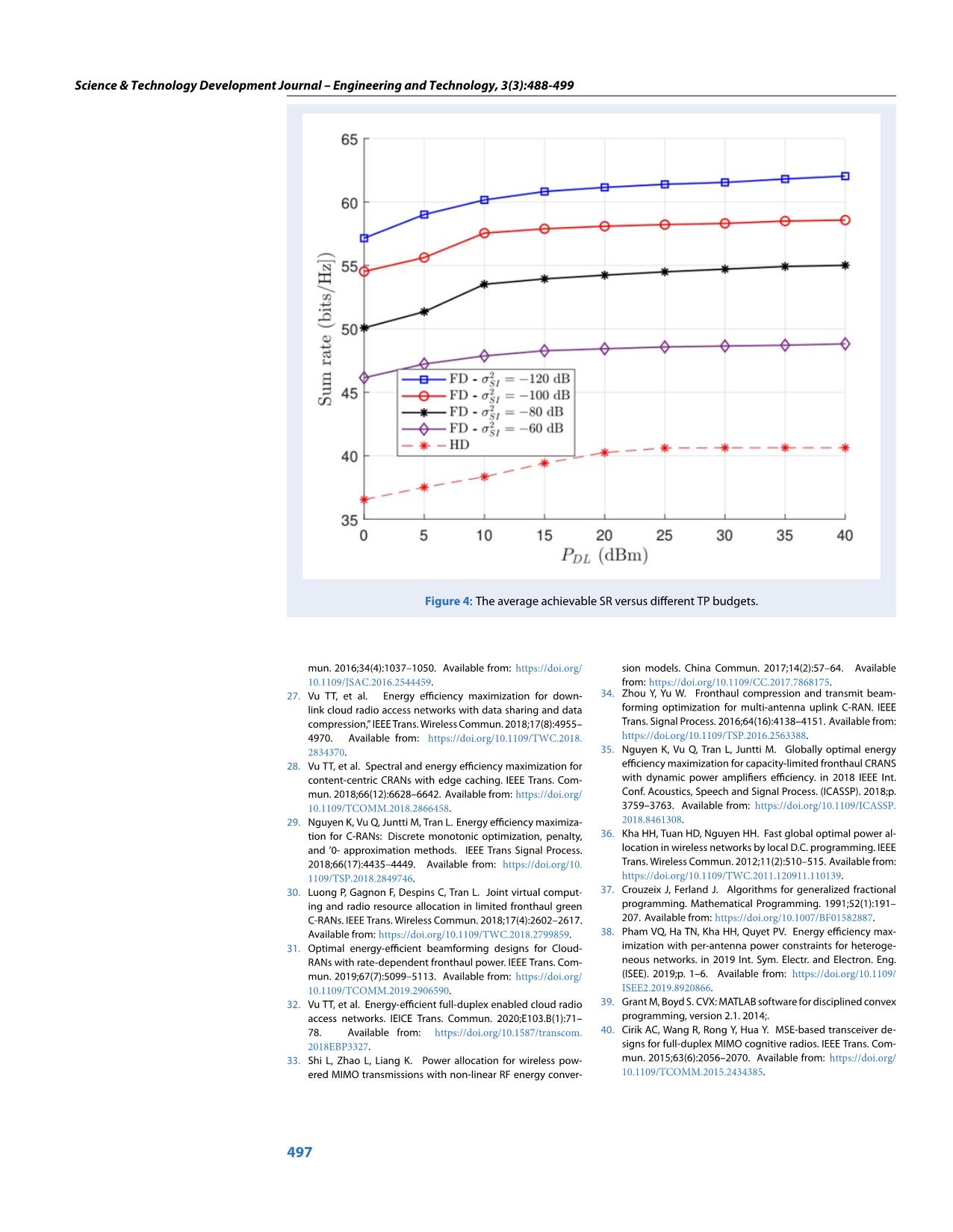
Trang 10
Tải về để xem bản đầy đủ
Tóm tắt nội dung tài liệu: Energy efficiency maximization for full duplex MIMO cloud radio access networks
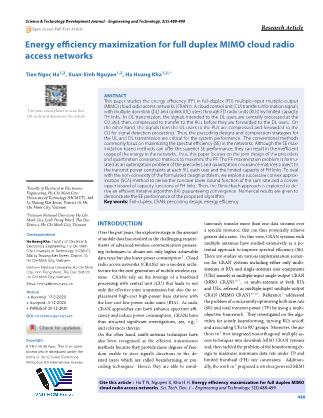
Science & Technology Development Journal – Engineering and Technology, 3(3):488-499 Open Access Full Text Article Research Article 1Faculty of Electrical & Electronics Engineering, Ho Chi Minh City University of Technology (HCMUT), 268 Ly Thuong Kiet Street, District 10, Ho Chi Minh City, Vietnam 2Vietnam National University Ho Chi Minh City, Linh Trung Ward, Thu Duc District, Ho Chi Minh City, Vietnam Correspondence Ha Hoang Kha, Faculty of Electrical & Electronics Engineering, Ho Chi Minh City University of Technology (HCMUT), 268 Ly Thuong Kiet Street, District 10, Ho Chi Minh City, Vietnam Vietnam National University Ho Chi Minh City, Linh Trung Ward, Thu Duc District, Ho Chi Minh City, Vietnam Email: hhkha@hcmut.edu.vn History Received: 17-3-2020 Accepted: 4-12-2020 Published: 20-12-2020 DOI :10.32508/stdjet.v3i3.685 Energy efficiencymaximization for full duplexMIMO cloud radio access networks Tien Ngoc Ha1,2, Xuan-Xinh Nguyen1,2, Ha Hoang Kha1,2,* Use your smartphone to scan this QR code and download this article ABSTRACT This paper studies the energy efficiency (EE) in full-duplex (FD) multiple-input multiple-output (MIMO) cloud radio access networks (CRANs). A cloud control unit (CU) transfers information signals with multiple downlink (DL) and uplink (UL) users through FD radio units (RUs) by limited capacity FH links. In DL transmission, the signals intended to the DL users are centrally processed at the CU and, then, compressed to transfer to the RUs before they are forwarded to the DL users. On the other hand, the signals from the UL users to the RUs are compressed and forwarded to the CU for signal detection processing. Thus, the precoding designs and compression strategies for the UL and DL transmission are critical for the system performance. The conventional methods commonly focus on maximizing the spectral efficiency (SE) in the networks. Although the SE max- imization based methods can offer the superior SE performance, they can result in the inefficient usage of the energy in the networks. Thus, this paper focuses on the joint design of the precoders and quantization covariance matrices to maximize the EE. The EE maximization problem is formu- lated as an optimization problem of the precoders and quantization covariancematrices subject to the transmit power constraints at each RU, each user and the limited capacity of FH links. To deal with the non-convexity of the formulated design problem, we exploit a successive convex approx- imation (SCA) method to derive the concave lower bound function of the sum rate and a convex upper bound of capacity functions of FH links. Then, the Dinkelbach approach is exploited to de- rive an efficient iterative algorithm (IA) guaranteeing convergence. Numerical results are given to demonstrate the EE performance of the proposed algorithm. Key words: Full-duplex, CRAN, precoding design, energy efficiency INTRODUCTION Over the past years, the explosive surge in the amount of mobile data has resulted in the challenging require- ments of advanced wireless communication possess- ing techniques to obtain not only higher achievable data rates but also lower power consumption1. Cloud radio access networks (CRANs) are a modern archi- tecture for the next generation of mobile wireless sys- tems. CRANs rely on the leverage of a baseband processing with central unit (CU) that leads to not only the effective joint transmission but also the re- placement high-cost high-power base stations with the low-cost low-power radio units (RUs). As such, CRAN approaches can both enhance spectrum effi- ciency and reduce power consumption. CRANs have thus attracted significant investigations, see, e.g., 2 and references therein. On the other hand, multi-antenna techniques have also been recognized as the efficient transmission methods because they provide more degrees of free- dom enable to steer signal’s directions to the de- sired users which are called beamforming or pre- coding techniques. Hence, they are able to simul- taneously transfer more than one data streams over a specific resource, that can thus potentially achieve greater data rates. On this view, CRAN systems with multiple antennas have studied extensively as a po- tential approach to improve spectral efficiency (SE). There are studies on various implementation scenar- ios for CRAN systems including either only multi- antenna at RUs and single-antenna user equipments (UEs) namely as multiple-input single-output CRAN (MISO CRAN)3–7, or multi-antenna at both RUs and UEs, referred as multiple-input multiple-output CRAN (MIMO CRAN) 8–10. Reference3 addressed the problemof concurrently optimizing both sum rate (SR) and total transmit power (TP) by using a multi- objective framework. They investigated on the algo- rithm for jointly beamforming, turning RUs on/off, and associating UEs to RU groups. Moreover, the au- thors in4 ... limitations are investigated in this example. In this ex- ample, the TP budgets of the RUs are set 25 dBm. The average achievable EE is depicted in Figure 5while the average achievable SR is shown in Figure 6. From the two figures, we can see that both achievable EE and SR are improved when the FH capacity increases. Noting that when the FH capacity is low, it is a bottleneck to restrict the system performance. In such cases, to re- duce the FH traffic, the system will switch to a single RU association19. Therefore, when the FH capacity increases, the achievable EE and SR are increased. On the other hand, the achievable EE and SR increase as SI decreases. CONCLUSION In this paper, a joint design of FH compression and precoding has been studied for a FD MIMO CRAN. We have addressed the EE maximization problem for this considered system model by developing the IA using successive convex approximation and Dinkel- bach approaches to deal with the non-convex prob- lem. Finally, by numerical simulation results, we have shown that the considered FDCRAN system can bring significant EE gains over the HD scheme when SI is low. In addition, the results have unveiled the interesting influence of FH rate levels on the system EE performance. Particularly, the bottleneck situa- tion caused by the limited FH capacity leads to the degradation in the EE system performance. ACKNOWLEDGMENT This research is funded by Vietnam National Foun- dation for Science and Technology Development (NAFOSTED) under grant number 102.04-2017.308. LIST OF ABBREVIATIONS CRAN: xloud radio access network CU: control unit D.C.: difference of convex DL: downlink EE: energy efficiency FD: full-duplex FH: fronthaul HD: half-duplex IA: iterative algorithm MIMO: multiple-input multiple-output MISO: multiple-input single-output OP: optimization problem QoS: quality-of-service RA: receive antenna RU: radio unit 494 Science & Technology Development Journal – Engineering and Technology, 3(3):488-499 Figure 2: Convergence behaviors of our IA for different levels of residual SI. SE: spectral efficiency SI: self-interference SR: sum-rate TA: transmit antenna TP: transmit power UE: user equipment UL: uplink CONFLICT OF INTEREST The authors declare that there is no conflict of interest regarding the publication of this article. AUTHORS’ CONTRIBUTIONS All the authors contribute equally to the paper includ- ing the research idea and written manuscript. REFERENCES 1. Singh K, Gupta A, Ratnarajah T, Ku M. A general approach toward green resource allocation in relay-assisted multiuser communication networks,” IEEE Trans. Wireless Commun. 2018;17(2):848–862. Available from: https://doi.org/10.1109/ TWC.2017.2772229. 2. PengM, Sun Y, Li X, Mao Z, Wang C. Recent advances in cloud radio access networks: System architectures, key techniques, and open issues. IEEE Commun. Surv. Tut. 2016;18(3):2282– 2308. Available from: https://doi.org/10.1109/COMST.2016. 2548658. 3. Luong P, Gagnon F, Despins C, Tran L. Optimal joint remote radio head selection and beamforming design for limited fronthaul CRAN. IEEE Trans. Signal Process. 2017;65(21):5605– 5620. Available from: https://doi.org/10.1109/TSP.2017. 2739102. 4. Lee S, Park S, Lee I. NOMA systems with content-centric mul- ticast transmission for C-RAN. IEEE Wireless Commun. Lett. 2018;7(5):828–831. Available from: https://doi.org/10.1109/ LWC.2018.2829514. 5. KimJ, LeeH, Park S, Lee I. Minimumratemaximization forwire- less powered cloud radio access networks. IEEE Trans. Veh. Technol. 2019;68(1):1045–1049. Available from: https://doi. org/10.1109/TVT.2018.2881969. 6. Kim J, Park S, Simeone O, Lee I, Shitz SS. Joint design of fron- thauling and hybrid beamforming for downlink C-RAN sys- tems. IEEE Trans. Commun. 2019;67(6):4423–4434. Available from: https://doi.org/10.1109/TCOMM.2019.2903142. 7. Yu D, Kim J, Park S. An efficient rate-splitting multiple ac- cess scheme for the downlink of C-RAN systems. IEEEWireless Commun Lett. 2019;8(6):1555–1558. Available from: https: //doi.org/10.1109/LWC.2019.2927206. 8. Park S, Simeone O, Sahin O, Shamai S. Joint precoding and multivariate backhaul compression for the downlink of cloud radio access networks. IEEE Trans. Signal Process. 2013;61(22):5646–5658. Available from: https://doi.org/10. 1109/TSP.2013.2280111. 9. Robust and efficient distributed compression for cloud ra- dio access networks. IEEE Trans. Veh. Technol. 2013;62(2):692– 703. Available from: https://doi.org/10.1109/TVT.2012. 2226945. 10. Inter-cluster design of precoding and fronthaul compression for cloud radio access networks. IEEE Wireless Commun. Lett. 495 Science & Technology Development Journal – Engineering and Technology, 3(3):488-499 Figure 3: The average achievable EE versus different TP budgets. 2014;3(4):369–372. Available from: https://doi.org/10.1109/ LWC.2014.2317735. 11. Zhang Z, Long K, Vasilakos AV, Hanzo L. Full-duplex wireless communications: Challenges, solutions, and future research directions. Proc. IEEE. 2016;104(7):1369–1409. Available from: https://doi.org/10.1109/JPROC.2015.2497203. 12. Sheng Z, Tuan HD, Tam HHM, Nguyen H. Energy efficient pre- coding in multicell networks with full-duplex base stations. EURASIP J. Wireless Commun. Network. 2017;48. Available from: https://doi.org/10.1186/s13638-017-0831-5. 13. Nguyen V, Duong TQ, Tuan HD, Shin O, Poor HV. Spec- tral and energy efficiencies in full-duplexwireless information and power transfer. IEEE Trans. Commun. 2017;65(5):2220– 2233. Available from: https://doi.org/10.1109/TCOMM.2017. 2665488. 14. Vu TT, Ngo DT, Ngo HQ, Le-Ngoc T. Full-duplex cell-free mas- sive MIMO. in ICC 2019 - 2019 IEEE Int. Conf. Commun. (ICC). 2019;p. 1–6. 15. Huberman S, Le-Ngoc T. Full-duplex MIMO precoding for sumrate maximization with sequential convex programming. IEEE Trans. Veh. Technol. 2015;64(11):5103–5112. Available from: https://doi.org/10.1109/TVT.2014.2380775. 16. Simeone O, Erkip E, Shamai S. Full-duplex cloud radio access networks: An information-theoretic viewpoint. IEEE Wireless Commun. Lett. 2014;3(4):413–416. Available from: https://doi. org/10.1109/LWC.2014.2323073. 17. Zhao M, Shi Q, Cai Y, Zhao M. Joint transceiver design for fullduplex cloud radio access networkswith SWIPT. IEEE Trans. Wireless Commun. 2017;16(9):5644–5658. Available from: https://doi.org/10.1109/TWC.2017.2712693. 18. Jeon Y, et al. Joint designs of fronthaul compression and precoding for full-duplex cloud radio access networks. IEEE Wireless Commun. Lett. 2016;5(6):632–635. Available from: https://doi.org/10.1109/LWC.2016.2609410. 19. Cirik AC, et al. Fronthaul compression and precoding de- sign for full-duplex cloud radio access network. IEEE Syst. J. 2019;13(2):1113–1124. Available from: https://doi.org/10. 1109/JSYST.2019.2900996. 20. Tang W, Feng S. User selection and power minimization in fullduplex cloud radio access networks. IEEE Trans. Signal Pro- cess. 2019;67(9):2426–2438. Available from: https://doi.org/ 10.1109/TSP.2019.2905804. 21. Fang C, Li P, Feng K. Joint interference cancellation and re- source allocation for full-duplex cloud radio access networks. IEEE Trans. Wireless Commun. 2019;18(6):3019–3033. Avail- able from: https://doi.org/10.1109/TWC.2019.2908173. 22. Buzzi S, et al. A survey of energy-efficient techniques for 5G networks and challenges ahead. IEEE J. Sel. Areas Commun. 2016;34(4):697–709. Available from: https://doi.org/10.1109/ JSAC.2016.2550338. 23. Sheng Z, et al. Power allocation for energy efficiency and secrecy of wireless interference networks. IEEE Trans. Wire- less Commun. 2018;17(6):3737–3751. Available from: https: //doi.org/10.1109/TWC.2018.2815626. 24. Nguyen X, Kha HH. Energy efficient full-duplex multicell mul- tiuserMIMOnetworks. in 2018 Int. Conf. Advan. Technol. Com- mun. (ATC). 2018;p. 163–167. Available from: https://doi.org/ 10.1109/ATC.2018.8587433. 25. Shi Y, Zhang J, Letaief KB. Group sparse beamform- ing for green Cloud-RAN. IEEE Trans. Wireless Commun. 2014;13(5):2809–2823. Available from: https://doi.org/10. 1109/TWC.2014.040214.131770. 26. Dai B, YuW. Energy efficiency of downlink transmission strate- gies for cloud radio access networks. IEEE J. Sel. Areas Com- 496 Science & Technology Development Journal – Engineering and Technology, 3(3):488-499 Figure 4: The average achievable SR versus different TP budgets. mun. 2016;34(4):1037–1050. Available from: https://doi.org/ 10.1109/JSAC.2016.2544459. 27. Vu TT, et al. Energy efficiency maximization for down- link cloud radio access networks with data sharing and data compression,” IEEE Trans.WirelessCommun. 2018;17(8):4955– 4970. Available from: https://doi.org/10.1109/TWC.2018. 2834370. 28. Vu TT, et al. Spectral and energy efficiency maximization for content-centric CRANs with edge caching. IEEE Trans. Com- mun. 2018;66(12):6628–6642. Available from: https://doi.org/ 10.1109/TCOMM.2018.2866458. 29. Nguyen K, Vu Q, Juntti M, Tran L. Energy efficiency maximiza- tion for C-RANs: Discrete monotonic optimization, penalty, and ’0- approximation methods. IEEE Trans Signal Process. 2018;66(17):4435–4449. Available from: https://doi.org/10. 1109/TSP.2018.2849746. 30. Luong P, Gagnon F, Despins C, Tran L. Joint virtual comput- ing and radio resource allocation in limited fronthaul green C-RANs. IEEE Trans. Wireless Commun. 2018;17(4):2602–2617. Available from: https://doi.org/10.1109/TWC.2018.2799859. 31. Optimal energy-efficient beamforming designs for Cloud- RANs with rate-dependent fronthaul power. IEEE Trans. Com- mun. 2019;67(7):5099–5113. Available from: https://doi.org/ 10.1109/TCOMM.2019.2906590. 32. Vu TT, et al. Energy-efficient full-duplex enabled cloud radio access networks. IEICE Trans. Commun. 2020;E103.B(1):71– 78. Available from: https://doi.org/10.1587/transcom. 2018EBP3327. 33. Shi L, Zhao L, Liang K. Power allocation for wireless pow- ered MIMO transmissions with non-linear RF energy conver- sion models. China Commun. 2017;14(2):57–64. Available from: https://doi.org/10.1109/CC.2017.7868175. 34. Zhou Y, Yu W. Fronthaul compression and transmit beam- forming optimization for multi-antenna uplink C-RAN. IEEE Trans. Signal Process. 2016;64(16):4138–4151. Available from: https://doi.org/10.1109/TSP.2016.2563388. 35. Nguyen K, Vu Q, Tran L, Juntti M. Globally optimal energy efficiency maximization for capacity-limited fronthaul CRANS with dynamic power amplifiers efficiency. in 2018 IEEE Int. Conf. Acoustics, Speech and Signal Process. (ICASSP). 2018;p. 3759–3763. Available from: https://doi.org/10.1109/ICASSP. 2018.8461308. 36. Kha HH, Tuan HD, Nguyen HH. Fast global optimal power al- location in wireless networks by local D.C. programming. IEEE Trans. Wireless Commun. 2012;11(2):510–515. Available from: https://doi.org/10.1109/TWC.2011.120911.110139. 37. Crouzeix J, Ferland J. Algorithms for generalized fractional programming. Mathematical Programming. 1991;52(1):191– 207. Available from: https://doi.org/10.1007/BF01582887. 38. Pham VQ, Ha TN, Kha HH, Quyet PV. Energy efficiency max- imization with per-antenna power constraints for heteroge- neous networks. in 2019 Int. Sym. Electr. and Electron. Eng. (ISEE). 2019;p. 1–6. Available from: https://doi.org/10.1109/ ISEE2.2019.8920866. 39. GrantM, Boyd S. CVX:MATLAB software for disciplined convex programming, version 2.1. 2014;. 40. Cirik AC, Wang R, Rong Y, Hua Y. MSE-based transceiver de- signs for full-duplex MIMO cognitive radios. IEEE Trans. Com- mun. 2015;63(6):2056–2070. Available from: https://doi.org/ 10.1109/TCOMM.2015.2434385. 497 Science & Technology Development Journal – Engineering and Technology, 3(3):488-499 Figure 5: The average achievable EE versus different levels ofCFH . 498 Figure 6: The average achievable SR versus different levels ofCFH . Tạp chí Phát triển Khoa học và Công nghệ – Kĩ thuật và Công nghệ, 3(3):488-499 Open Access Full Text Article Bài Nghiên cứu 1Khoa Điện-Điện tử, Trường Đại học Bách Khoa TP.HCM, Việt Nam 2Đại học Quốc gia Thành phố Hồ Chí Minh Liên hệ Hà Hoàng Kha, Khoa Điện-Điện tử, Trường Đại học Bách Khoa TP.HCM, Việt Nam Đại học Quốc gia Thành phố Hồ Chí Minh Email: hhkha@hcmut.edu.vn Lịch sử Ngày nhận: 17-3-2020 Ngày chấp nhận: 4-12-2020 Ngày đăng: 20-12-2020 DOI :10.32508/stdjet.v3i3.685 Bản quyền © ĐHQG Tp.HCM. Đây là bài báo công bố mở được phát hành theo các điều khoản của the Creative Commons Attribution 4.0 International license. Cực đại hóa hiệu suất năng lượng trong hệ thốngMIMO CRAN song công Hà Ngọc Tiến1,2, Nguyễn Xuân Xinh1,2, Hà Hoàng Kha1,2,* Use your smartphone to scan this QR code and download this article TÓM TẮT Bài báo này nghiên cứu hiệu quả năng lượng của hệ thốngMIMO CRAN song công. Một trạm điều khiển trung tâm (CU) truyền tín hiệu thông tin với các thiết bị người dùng đường lên và đường xuống thông qua các trạm vô tuyến RU song công bằng các đường liên kết FH có dung lượng giới hạn. Trong kênh truyền đường xuống, các tín hiệu mong muốn phát cho người dùng đường xuống được xử lý tập trung ở trạm điều khiển trung tâm và sau đó được nén để truyền đến các trạm vô tuyến RU trước khi chúng được chuyển tiếp đến người dùng đường xuống. Mặc khác, các tín hiệu từ người dung đường lên đến các trạm vô tuyến được nén và chuyển đến trạm điều khiển trung tâm cho việc xử lý giải mã tín hiệu. Do đó, việc thiết kế tiền mã hóa và chiến lược nén cho truyền đường lên và đường xuống là quan trọng đối với hiệu năng hệ thống. Các phương pháp phổ biến là tập trung tối đa hóa hiệu suất phổ trong mạng. Mặc dù các phương pháp dựa trên cực đại hóa hiệu suất phổ có thể cung cấp hiệu suất phổ tốt, chúng có thể dẫn đến việc sử dụng năng lượng không hiệu quả. Do đó, bài báo này tập trung thiết kế đồng thời bộ tiền mã hóa và các ma trận hiệp phương sai lượng tử để tối đa hóa hiệu suất năng lượng. Vấn đề tối đa hóa hiệu suất năng lượng được biểu diễn như một bài toán tối ưu của các ma trận tiền mã hóa và ma trân hiệu phương sai lượng tử phụ thuộc vào điều kiện ràng buộc công suất phát ở mỗi trạm vô tuyến, mỗi người dùng và dung lượng giới hạn của các liên kết FH. Để giải quyết vấn đề không lồi của bài toán thiết kế, chúng tôi khai thác phương pháp xấp xỉ lồi tuần tự để rút ra hàm biên dưới lõm của tổng tốc độ kênh và biên trên lồi của dung lượng đường liên kế FH. Sau đó, giải thuật Dinkelbach được khai thác để phát triển giải thuật lặp hiệu quả đảm bảo tính hội tụ. Các kết quả mô phỏng số được thực hiện để minh họa hiệu suất năng lượng EE của giải thuật. Từ khoá: Song công, CRAN, thiết kế tiền mã hóa, hiệu suất năng lượng Trích dẫn bài báo này: Tiến H N, Xinh N X, Kha H H. Cực đại hóa hiệu suất năng lượng trong hệ thống MIMO CRAN song công. Sci. Tech. Dev. J. - Eng. Tech.; 3(3):488-499. 499
File đính kèm:
energy_efficiency_maximization_for_full_duplex_mimo_cloud_ra.pdf