A prediction model of road traffic fatalities in Ha Noi using improved grey model GM (1,1)
Abstract: This study improves the first-order and one-variable grey differential equation
model (abbreviated as GM(1,1) model), which requires only a limited amount of data to
estimate the behavior of a system with unknown and known information, to predict road traffic
fatalities (RTFs) in Hanoi in short-term with experimental data of RTFs from 2010 to 2018.
Firstly, we introduce basic concept of original GM(1,1), and then an improved GM(1,1) model
is established based on the modification of the errors. Finally, we compare the performance of
forecasting models to predict RTFs in Hanoi. The results of prediction models show that the
forecasting accuracy of the improved GM(1,1) model is higher than the original GM(1,1)
model. Comparison with original GM(1,1) model, mean absolute percentage error (MAPE) of
the improved GM(1,1) model is considerably decreased from 8.30% to 1.70%. This proves the
applicability of the improved GM(1,1) model to make a short-term prediction for RTFs in
Hanoi.
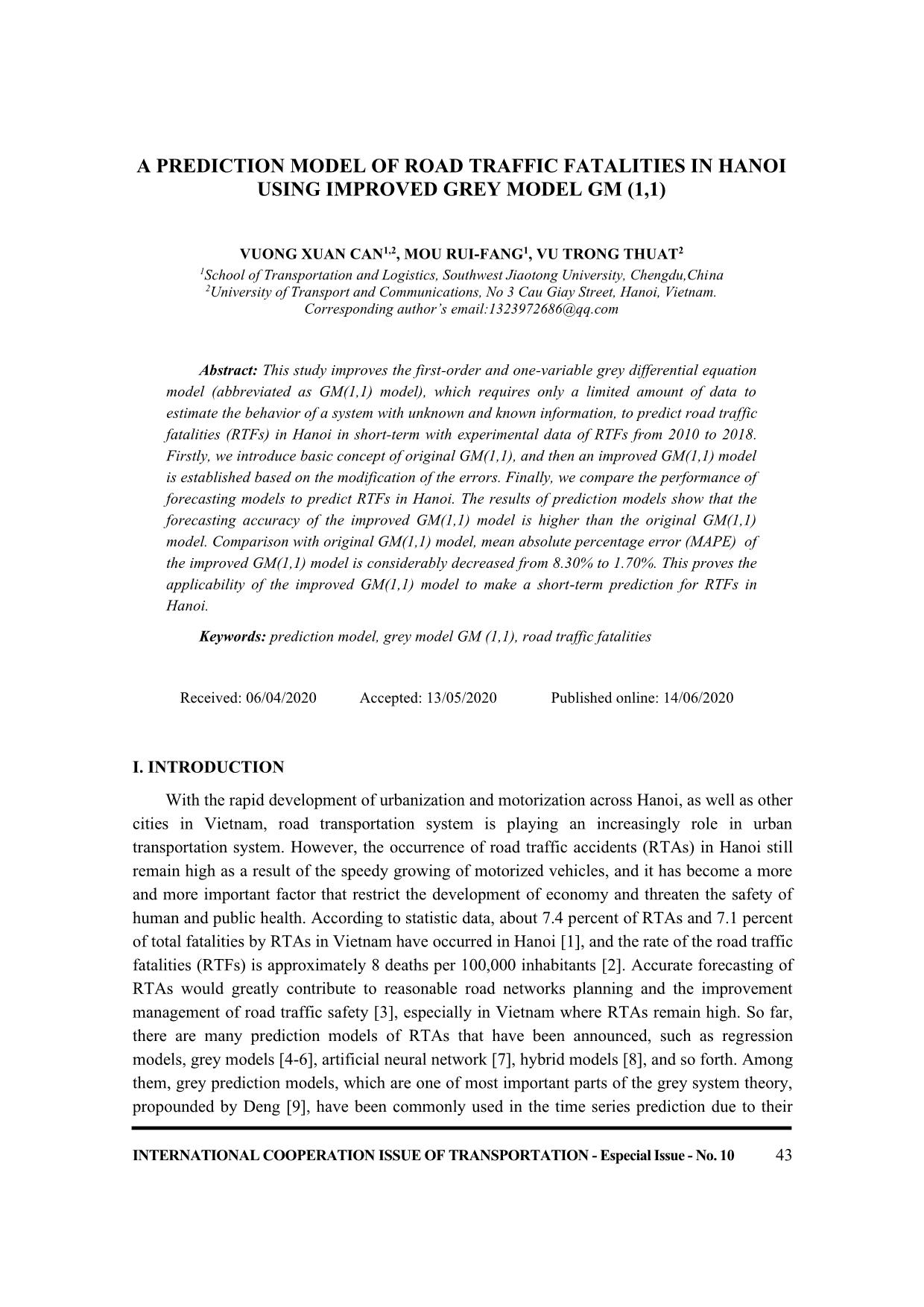
Trang 1
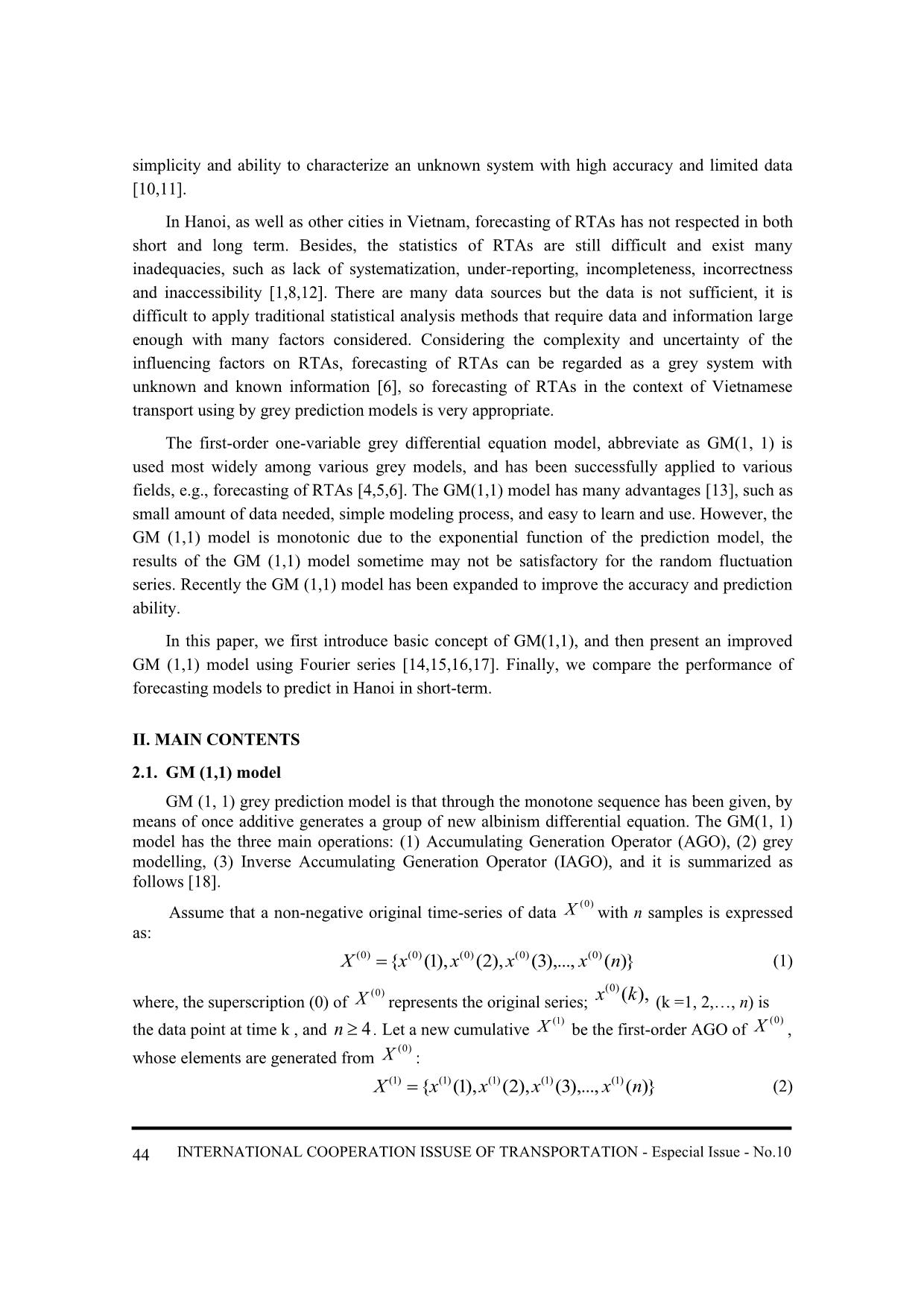
Trang 2
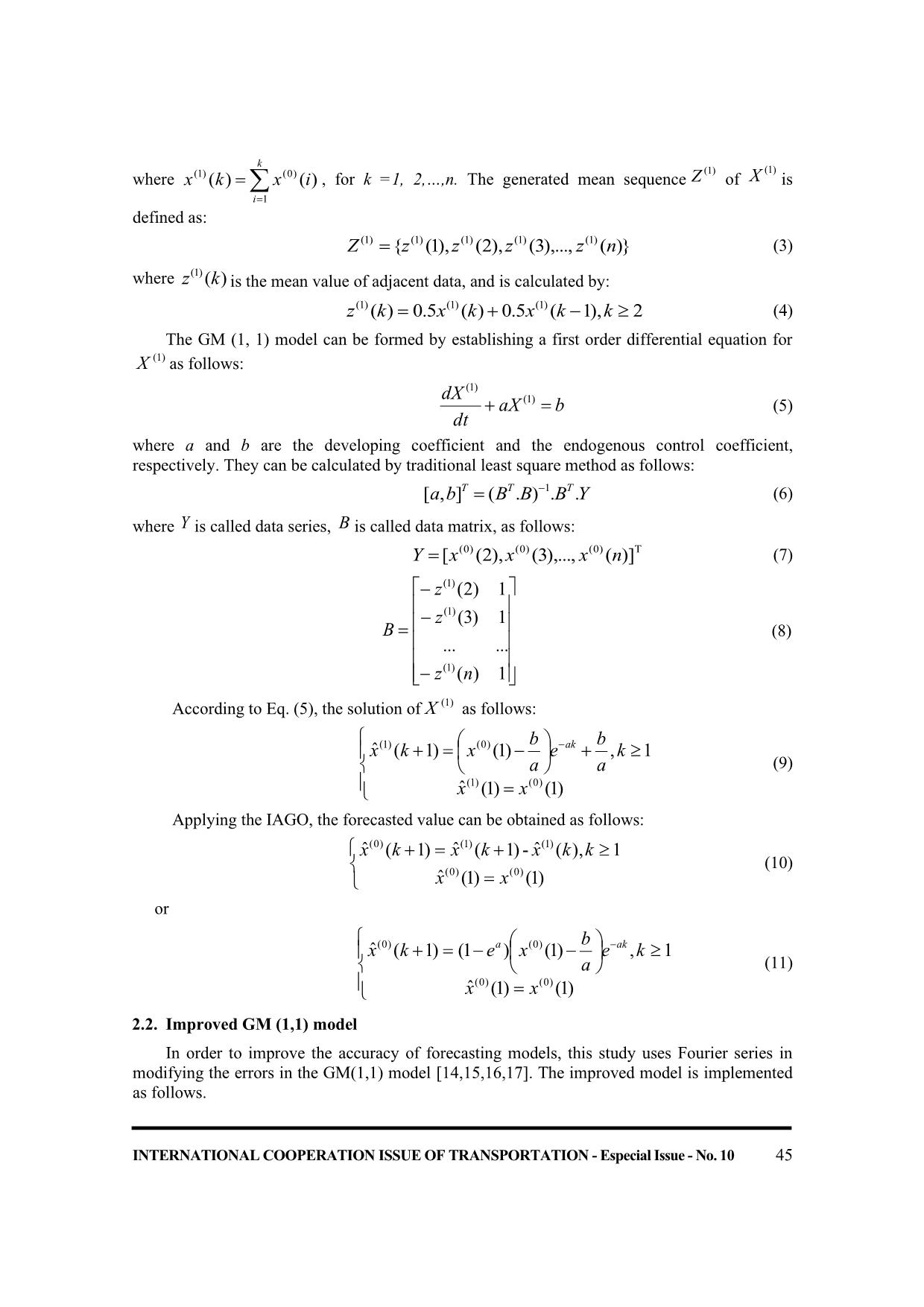
Trang 3
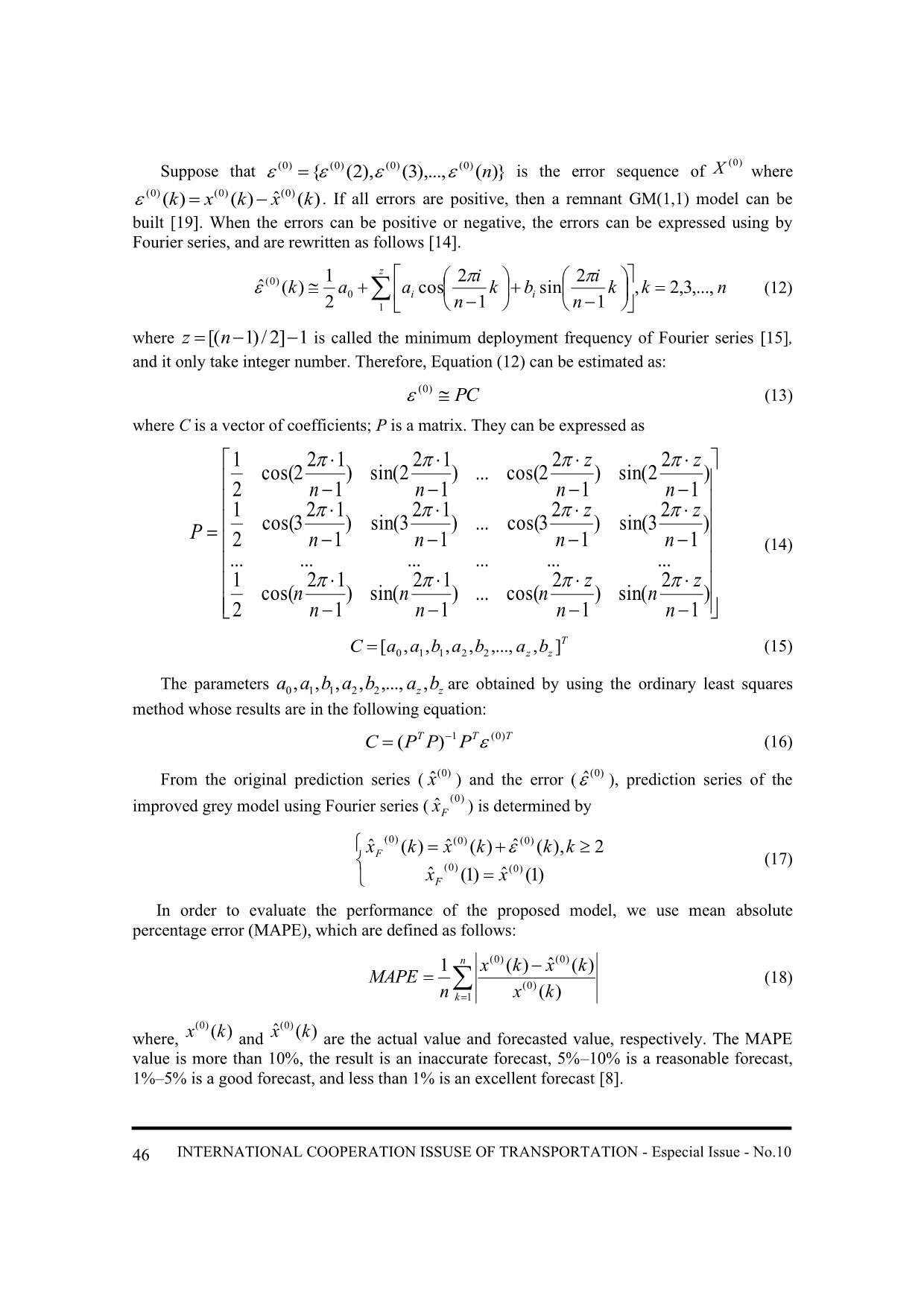
Trang 4
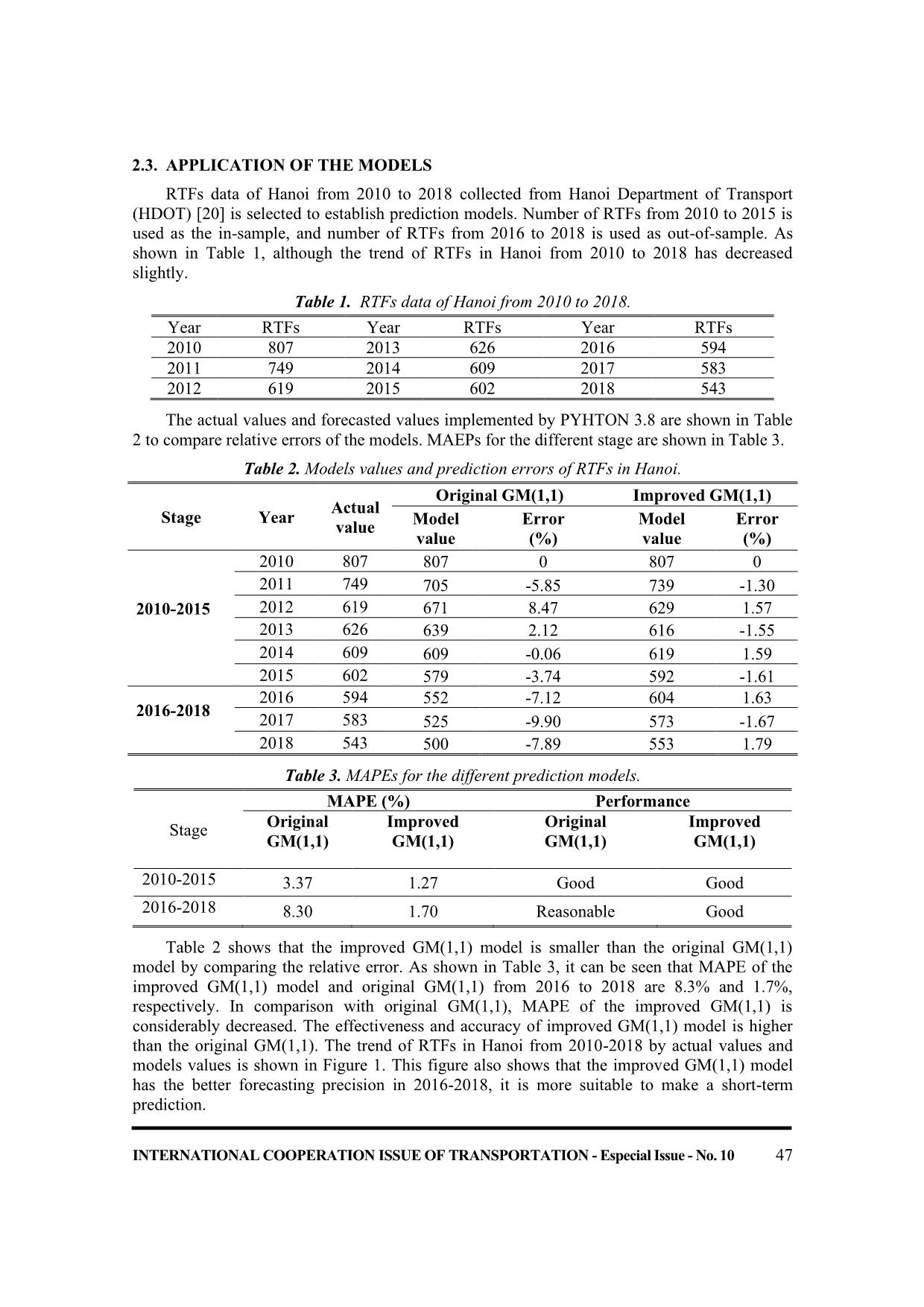
Trang 5
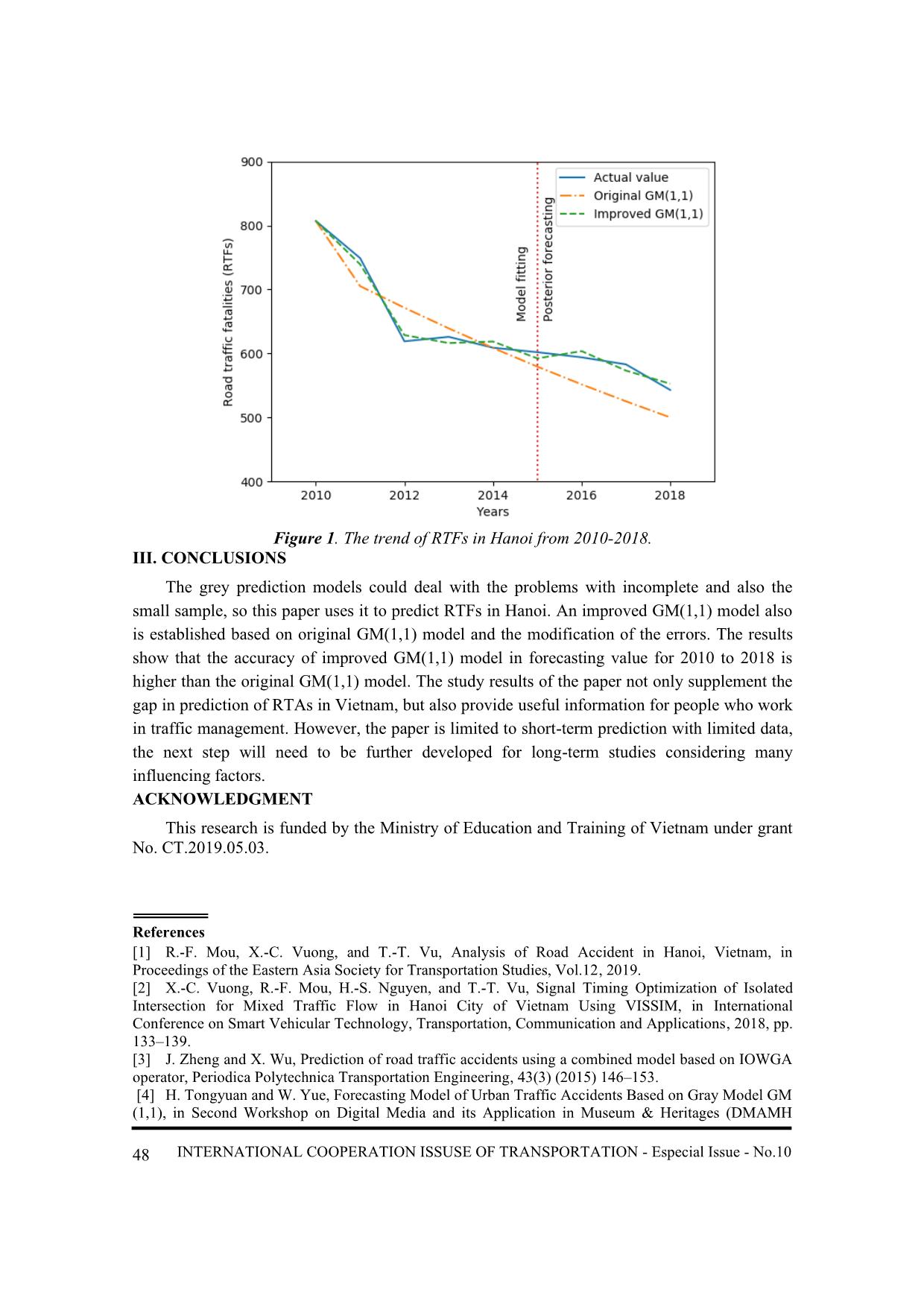
Trang 6
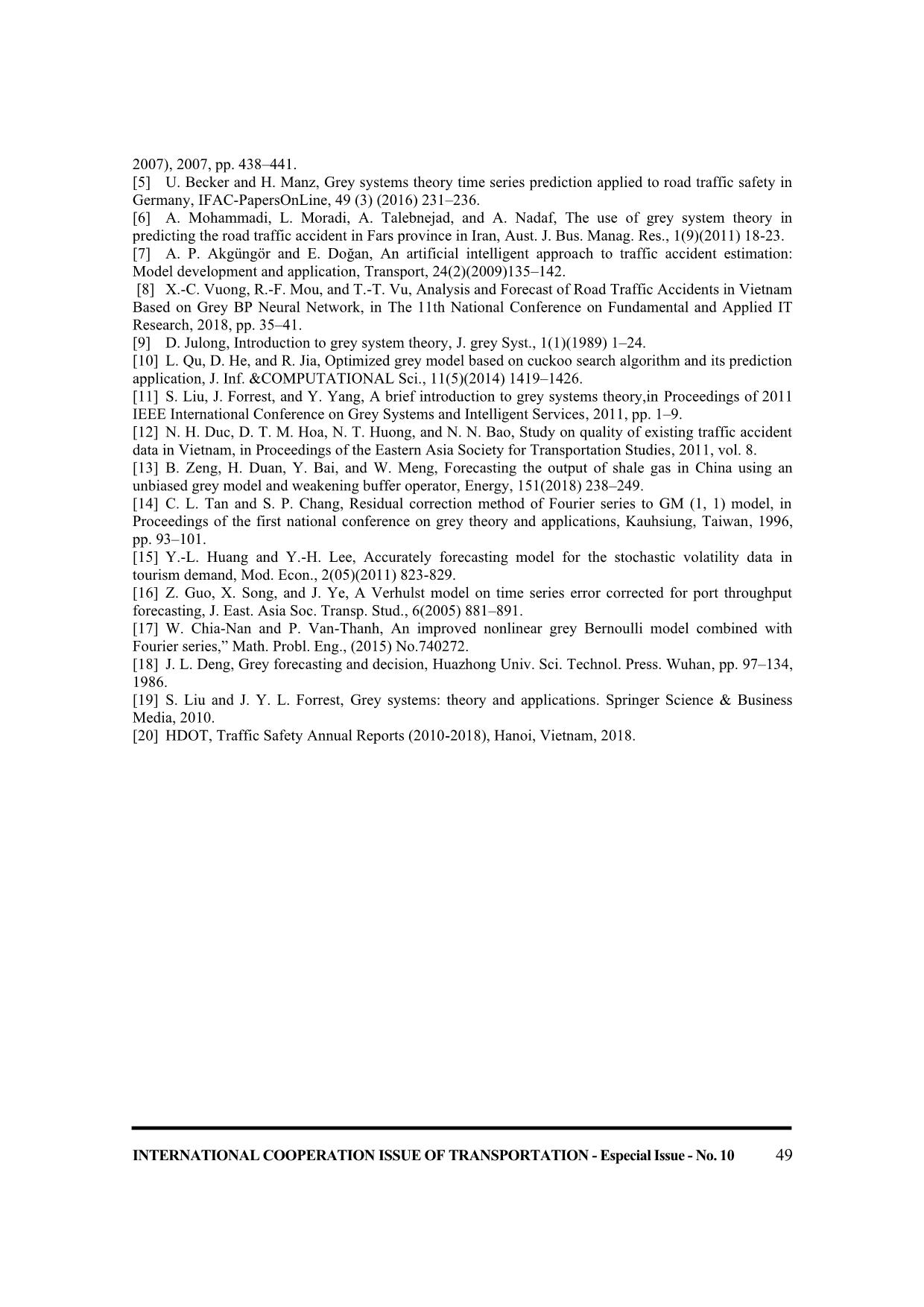
Trang 7
Tóm tắt nội dung tài liệu: A prediction model of road traffic fatalities in Ha Noi using improved grey model GM (1,1)
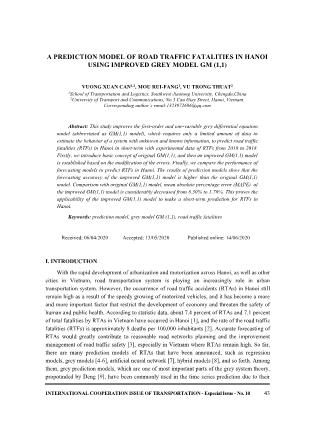
INTERNATIONAL COOPERATION ISSUE OF TRANSPORTATION - Especial Issue - No. 10 43 I. INTRODUCTION With the rapid development of urbanization and motorization across Hanoi, as well as other cities in Vietnam, road transportation system is playing an increasingly role in urban transportation system. However, the occurrence of road traffic accidents (RTAs) in Hanoi still remain high as a result of the speedy growing of motorized vehicles, and it has become a more and more important factor that restrict the development of economy and threaten the safety of human and public health. According to statistic data, about 7.4 percent of RTAs and 7.1 percent of total fatalities by RTAs in Vietnam have occurred in Hanoi [1], and the rate of the road traffic fatalities (RTFs) is approximately 8 deaths per 100,000 inhabitants [2]. Accurate forecasting of RTAs would greatly contribute to reasonable road networks planning and the improvement management of road traffic safety [3], especially in Vietnam where RTAs remain high. So far, there are many prediction models of RTAs that have been announced, such as regression models, grey models [4-6], artificial neural network [7], hybrid models [8], and so forth. Among them, grey prediction models, which are one of most important parts of the grey system theory, propounded by Deng [9], have been commonly used in the time series prediction due to their A PREDICTION MODEL OF ROAD TRAFFIC FATALITIES IN HANOI USING IMPROVED GREY MODEL GM (1,1) VUONG XUAN CAN1,2, MOU RUI-FANG1, VU TRONG THUAT2 1School of Transportation and Logistics, Southwest Jiaotong University, Chengdu,China 2University of Transport and Communications, No 3 Cau Giay Street, Hanoi, Vietnam. Corresponding author’s email:1323972686@qq.com Abstract: This study improves the first-order and one-variable grey differential equation model (abbreviated as GM(1,1) model), which requires only a limited amount of data to estimate the behavior of a system with unknown and known information, to predict road traffic fatalities (RTFs) in Hanoi in short-term with experimental data of RTFs from 2010 to 2018. Firstly, we introduce basic concept of original GM(1,1), and then an improved GM(1,1) model is established based on the modification of the errors. Finally, we compare the performance of forecasting models to predict RTFs in Hanoi. The results of prediction models show that the forecasting accuracy of the improved GM(1,1) model is higher than the original GM(1,1) model. Comparison with original GM(1,1) model, mean absolute percentage error (MAPE) of the improved GM(1,1) model is considerably decreased from 8.30% to 1.70%. This proves the applicability of the improved GM(1,1) model to make a short-term prediction for RTFs in Hanoi. Keywords: prediction model, grey model GM (1,1), road traffic fatalities Received: 06/04/2020 Accepted: 13/05/2020 Published online: 14/06/2020 INTERNATIONAL COOPERATION ISSUSE OF TRANSPORTATION - Especial Issue - No.10 44 simplicity and ability to characterize an unknown system with high accuracy and limited data [10,11]. In Hanoi, as well as other cities in Vietnam, forecasting of RTAs has not respected in both short and long term. Besides, the statistics of RTAs are still difficult and exist many inadequacies, such as lack of systematization, under-reporting, incompleteness, incorrectness and inaccessibility [1,8,12]. There are many data sources but the data is not sufficient, it is difficult to apply traditional statistical analysis methods that require data and information large enough with many factors considered. Considering the complexity and uncertainty of the influencing factors on RTAs, forecasting of RTAs can be regarded as a grey system with unknown and known information [6], so forecasting of RTAs in the context of Vietnamese transport using by grey prediction models is very appropriate. The first-order one-variable grey differential equation model, abbreviate as GM(1, 1) is used most widely among various grey models, and has been successfully applied to various fields, e.g., forecasting of RTAs [4,5,6]. The GM(1,1) model has many advantages [13], such as small amount of data needed, simple modeling process, and easy to learn and use. However, the GM (1,1) model is monotonic due to the exponential function of the prediction model, the results of the GM (1,1) model sometime may not be satisfactory for the random fluctuation series. Recently the GM (1,1) model has been expanded to improve the accuracy and prediction ability. In this paper, we first introduce basic concept of GM(1,1), and then present an improved GM (1,1) model using Fourier series [14,15,16,17]. Finally, we compare the performance of forecasting models to predict in Hanoi in short-term. II. MAIN CONTENTS 2.1. GM (1,1) model GM (1, 1) grey prediction model is that through the monotone sequence has been given, by means of once additive generates a group of new albinism differential equation. The GM(1, 1) model has the three main operations: (1) Accumulating Generation Operator (AGO), (2) grey modelling, (3) Inverse Accumulating Generation Operator (IAGO), and it is summarized as follows [18]. Assume that a non-negative original time-series of data )0(X with n samples is expressed as: })(),...,3(),2(),1({ )0()0()0()0()0( nxxxxX = (1) where, the superscription (0) of )0(X represents the original series; ),( )0( kx (k =1, 2,, n) is the data point at time k , and 4 n . Let a new cumulative )1(X be the first-order AGO of )0(X , whose elements are generated from )0(X : })(),...,3(),2(),1({ )1()1()1()1()1( nxxxxX = (2) INTERNATIONAL COOPERATION ISSUE OF TRANSPORTATION - Especial Issue - No. 10 45 where = = k i ixkx 1 )0()1( )()( , for k =1, 2,,n. The generated mean sequence )1(Z of )1(X is defined as: })(),...,3(),2(),1({ )1()1()1()1()1( nzzzzZ = (3) where )()1( kz is the mean value of adjacent data, and is calculated by: 2),1(5.0)(5.0)( )1()1()1( −+= kkxkxkz (4) The GM (1, 1) model can be formed by establishing a first order differential equation for )1(X as follows: baX dt dX =+ )1( )1( (5) where a and b are the developing coefficient and the endogenous control coefficient, respectively. They can be calculated by traditional least square method as follows: YBBBba TTT ..).(],[ 1−= (6) where Y is called data series, B is called data matrix, as follows: T)0()0()0( ])(),...,3(),2([ nxxxY = (7) − − − = 1)( ...... 1)3( 1)2( )1( )1( )1( nz z z B (8) According to Eq. (5), the solution of )1(X as follows: = + −=+ − )1()1(ˆ 1,)1()1(ˆ )0()1( )0()1( xx k a b e a b xkx ak (9) Applying the IAGO, the forecasted value can be obtained as follows: = +=+ )1()1(ˆ 1),(ˆ-)1(ˆ)1(ˆ )0()0( )1()1()0( xx kkxkxkx (10) or = −−=+ − )1()1(ˆ 1,)1()1()1(ˆ )0()0( )0()0( xx ke a b xekx aka (11) 2.2. Improved GM (1,1) model In order to improve the accuracy of forecasting models, this study uses Fourier series in modifying the errors in the GM(1,1) model [14,15,16,17]. The improved model is implemented as follows. INTERNATIONAL COOPERATION ISSUSE OF TRANSPORTATION - Especial Issue - No.10 46 Suppose that })(),...,3(),2({ )0()0()0()0( n = is the error sequence of )0(X where )(ˆ)()( )0()0()0( kxkxk −= . If all errors are positive, then a remnant GM(1,1) model can be built [19]. When the errors can be positive or negative, the errors can be expressed using by Fourier series, and are rewritten as follows [14]. nkk n i bk n i aak z ii ,...,3,2, 1 2 sin 1 2 cos 2 1 )(ˆ 1 0 )0( = − + − + (12) where 1]2/)1[( −−= nz is called the minimum deployment frequency of Fourier series [15], and it only take integer number. Therefore, Equation (12) can be estimated as: PC)0( (13) where C is a vector of coefficients; P is a matrix. They can be expressed as − − − − − − − − − − − − = ) 1 2 sin() 1 2 cos(...) 1 12 sin() 1 12 cos( 2 1 .................. ) 1 2 3sin() 1 2 3cos(...) 1 12 3sin() 1 12 3cos( 2 1 ) 1 2 2sin() 1 2 2cos(...) 1 12 2sin() 1 12 2cos( 2 1 n z n n z n n n n n n z n z nn n z n z nn P (14) T zz bababaaC ],,...,,,,,[ 22110= (15) The parameters zz bababaa ,,...,,,,, 22110 are obtained by using the ordinary least squares method whose results are in the following equation: TTT PPPC )0(1)( −= (16) From the original prediction series ( )0(xˆ ) and the error ( )0(ˆ ), prediction series of the improved grey model using Fourier series ( )0( ˆ Fx ) is determined by = += )1(ˆ)1(ˆ 2),(ˆ)(ˆ)(ˆ )0()0( )0()0()0( xx kkkxkx F F (17) In order to evaluate the performance of the proposed model, we use mean absolute percentage error (MAPE), which are defined as follows: = − = n k kx kxkx n MAPE 1 )0( )0()0( )( )(ˆ)(1 (18) where, )( )0( kx and )(ˆ )0( kx are the actual value and forecasted value, respectively. The MAPE value is more than 10%, the result is an inaccurate forecast, 5%–10% is a reasonable forecast, 1%–5% is a good forecast, and less than 1% is an excellent forecast [8]. INTERNATIONAL COOPERATION ISSUE OF TRANSPORTATION - Especial Issue - No. 10 47 2.3. APPLICATION OF THE MODELS RTFs data of Hanoi from 2010 to 2018 collected from Hanoi Department of Transport (HDOT) [20] is selected to establish prediction models. Number of RTFs from 2010 to 2015 is used as the in-sample, and number of RTFs from 2016 to 2018 is used as out-of-sample. As shown in Table 1, although the trend of RTFs in Hanoi from 2010 to 2018 has decreased slightly. Table 1. RTFs data of Hanoi from 2010 to 2018. Year RTFs Year RTFs Year RTFs 2010 807 2013 626 2016 594 2011 749 2014 609 2017 583 2012 619 2015 602 2018 543 The actual values and forecasted values implemented by PYHTON 3.8 are shown in Table 2 to compare relative errors of the models. MAEPs for the different stage are shown in Table 3. Table 2. Models values and prediction errors of RTFs in Hanoi. Stage Year Actual value Original GM(1,1) Improved GM(1,1) Model value Error (%) Model value Error (%) 2010-2015 2010 807 807 0 807 0 2011 749 705 -5.85 739 -1.30 2012 619 671 8.47 629 1.57 2013 626 639 2.12 616 -1.55 2014 609 609 -0.06 619 1.59 2015 602 579 -3.74 592 -1.61 2016-2018 2016 594 552 -7.12 604 1.63 2017 583 525 -9.90 573 -1.67 2018 543 500 -7.89 553 1.79 Table 3. MAPEs for the different prediction models. Stage MAPE (%) Performance Original GM(1,1) Improved GM(1,1) Original GM(1,1) Improved GM(1,1) 2010-2015 3.37 1.27 Good Good 2016-2018 8.30 1.70 Reasonable Good Table 2 shows that the improved GM(1,1) model is smaller than the original GM(1,1) model by comparing the relative error. As shown in Table 3, it can be seen that MAPE of the improved GM(1,1) model and original GM(1,1) from 2016 to 2018 are 8.3% and 1.7%, respectively. In comparison with original GM(1,1), MAPE of the improved GM(1,1) is considerably decreased. The effectiveness and accuracy of improved GM(1,1) model is higher than the original GM(1,1). The trend of RTFs in Hanoi from 2010-2018 by actual values and models values is shown in Figure 1. This figure also shows that the improved GM(1,1) model has the better forecasting precision in 2016-2018, it is more suitable to make a short-term prediction. INTERNATIONAL COOPERATION ISSUSE OF TRANSPORTATION - Especial Issue - No.10 48 Figure 1. The trend of RTFs in Hanoi from 2010-2018. III. CONCLUSIONS The grey prediction models could deal with the problems with incomplete and also the small sample, so this paper uses it to predict RTFs in Hanoi. An improved GM(1,1) model also is established based on original GM(1,1) model and the modification of the errors. The results show that the accuracy of improved GM(1,1) model in forecasting value for 2010 to 2018 is higher than the original GM(1,1) model. The study results of the paper not only supplement the gap in prediction of RTAs in Vietnam, but also provide useful information for people who work in traffic management. However, the paper is limited to short-term prediction with limited data, the next step will need to be further developed for long-term studies considering many influencing factors. ACKNOWLEDGMENT This research is funded by the Ministry of Education and Training of Vietnam under grant No. CT.2019.05.03. References [1] R.-F. Mou, X.-C. Vuong, and T.-T. Vu, Analysis of Road Accident in Hanoi, Vietnam, in Proceedings of the Eastern Asia Society for Transportation Studies, Vol.12, 2019. [2] X.-C. Vuong, R.-F. Mou, H.-S. Nguyen, and T.-T. Vu, Signal Timing Optimization of Isolated Intersection for Mixed Traffic Flow in Hanoi City of Vietnam Using VISSIM, in International Conference on Smart Vehicular Technology, Transportation, Communication and Applications, 2018, pp. 133–139. [3] J. Zheng and X. Wu, Prediction of road traffic accidents using a combined model based on IOWGA operator, Periodica Polytechnica Transportation Engineering, 43(3) (2015) 146–153. [4] H. Tongyuan and W. Yue, Forecasting Model of Urban Traffic Accidents Based on Gray Model GM (1,1), in Second Workshop on Digital Media and its Application in Museum & Heritages (DMAMH INTERNATIONAL COOPERATION ISSUE OF TRANSPORTATION - Especial Issue - No. 10 49 2007), 2007, pp. 438–441. [5] U. Becker and H. Manz, Grey systems theory time series prediction applied to road traffic safety in Germany, IFAC-PapersOnLine, 49 (3) (2016) 231–236. [6] A. Mohammadi, L. Moradi, A. Talebnejad, and A. Nadaf, The use of grey system theory in predicting the road traffic accident in Fars province in Iran, Aust. J. Bus. Manag. Res., 1(9)(2011) 18-23. [7] A. P. Akgüngör and E. Doğan, An artificial intelligent approach to traffic accident estimation: Model development and application, Transport, 24(2)(2009)135–142. [8] X.-C. Vuong, R.-F. Mou, and T.-T. Vu, Analysis and Forecast of Road Traffic Accidents in Vietnam Based on Grey BP Neural Network, in The 11th National Conference on Fundamental and Applied IT Research, 2018, pp. 35–41. [9] D. Julong, Introduction to grey system theory, J. grey Syst., 1(1)(1989) 1–24. [10] L. Qu, D. He, and R. Jia, Optimized grey model based on cuckoo search algorithm and its prediction application, J. Inf. &COMPUTATIONAL Sci., 11(5)(2014) 1419–1426. [11] S. Liu, J. Forrest, and Y. Yang, A brief introduction to grey systems theory,in Proceedings of 2011 IEEE International Conference on Grey Systems and Intelligent Services, 2011, pp. 1–9. [12] N. H. Duc, D. T. M. Hoa, N. T. Huong, and N. N. Bao, Study on quality of existing traffic accident data in Vietnam, in Proceedings of the Eastern Asia Society for Transportation Studies, 2011, vol. 8. [13] B. Zeng, H. Duan, Y. Bai, and W. Meng, Forecasting the output of shale gas in China using an unbiased grey model and weakening buffer operator, Energy, 151(2018) 238–249. [14] C. L. Tan and S. P. Chang, Residual correction method of Fourier series to GM (1, 1) model, in Proceedings of the first national conference on grey theory and applications, Kauhsiung, Taiwan, 1996, pp. 93–101. [15] Y.-L. Huang and Y.-H. Lee, Accurately forecasting model for the stochastic volatility data in tourism demand, Mod. Econ., 2(05)(2011) 823-829. [16] Z. Guo, X. Song, and J. Ye, A Verhulst model on time series error corrected for port throughput forecasting, J. East. Asia Soc. Transp. Stud., 6(2005) 881–891. [17] W. Chia-Nan and P. Van-Thanh, An improved nonlinear grey Bernoulli model combined with Fourier series,” Math. Probl. Eng., (2015) No.740272. [18] J. L. Deng, Grey forecasting and decision, Huazhong Univ. Sci. Technol. Press. Wuhan, pp. 97–134, 1986. [19] S. Liu and J. Y. L. Forrest, Grey systems: theory and applications. Springer Science & Business Media, 2010. [20] HDOT, Traffic Safety Annual Reports (2010-2018), Hanoi, Vietnam, 2018.
File đính kèm:
a_prediction_model_of_road_traffic_fatalities_in_ha_noi_usin.pdf